Principles of Neural Coding
In addition, the book describes alternative approaches based on simulations with neural networks and in silico modeling in this highly interdisciplinary topic. It can serve as an important reference to students and professionals. He holds a research chair and is the director of the Centre for Systems Neuroscience and the head of the Bioengineering Research Group at the University of Leicester.
Guidelines for Paper Presentations:
His main research interest is on the study of the principles of visual perception and memory. Together with colleagues at Caltech and UCLA, he discovered what has been named "Concept cells" or "Jennifer Aniston neurons"—neurons in the human brain that play a key role in memory formation. He has worked as senior scientist at the Italian Institute of Technology since and as chair in the Formal Analysis of Cortical Networks at the University of Glasgow since We provide complimentary e-inspection copies of primary textbooks to instructors considering our books for course adoption.
Learn More about VitalSource Bookshelf. CPD consists of any educational activity which helps to maintain and develop knowledge, problem-solving, and technical skills with the aim to provide better health care through higher standards. It could be through conference attendance, group discussion or directed reading to name just a few examples. We provide a free online form to document your learning and a certificate for your records. Already read this title?
Principles of neural coding.
Please accept our apologies for any inconvenience this may cause. Exclusive web offer for individuals. Add to Wish List. Toggle navigation Additional Book Information. Description Table of Contents Editor s Bio. Summary Understanding how populations of neurons encode information is the challenge faced by researchers in the field of neural coding. Provides a comprehensive and interdisciplinary approach Describes topics of interest to a wide range of researchers The book then moves forward with the description of the principles of neural coding for different functions and in different species and concludes with theoretical and modeling works describing how information processing functions are implemented.
Biophysical Origin and Analysis Gaute T. The specificity of temporal coding requires highly refined technology to measure informative, reliable, experimental data. Advances made in optogenetics allow neurologists to control spikes in individual neurons, offering electrical and spatial single-cell resolution. For example, blue light causes the light-gated ion channel channelrhodopsin to open, depolarizing the cell and producing a spike. When blue light is not sensed by the cell, the channel closes, and the neuron ceases to spike. The pattern of the spikes matches the pattern of the blue light stimuli.
By inserting channelrhodopsin gene sequences into mouse DNA, researchers can control spikes and therefore certain behaviors of the mouse e. Optogenetic technology also has the potential to enable the correction of spike abnormalities at the root of several neurological and psychological disorders. Regulation of spike intervals in single cells more precisely controls brain activity than the addition of pharmacological agents intravenously.
Phase-of-firing code is a neural coding scheme that combines the spike count code with a time reference based on oscillations. This type of code takes into account a time label for each spike according to a time reference based on phase of local ongoing oscillations at low [41] or high frequencies. It has been shown that neurons in some cortical sensory areas encode rich naturalistic stimuli in terms of their spike times relative to the phase of ongoing network oscillatory fluctuations, rather than only in terms of their spike count.
The phase-of-firing code is often categorized as a temporal code although the time label used for spikes i. As a result, often only four discrete values for the phase are enough to represent all the information content in this kind of code with respect to the phase of oscillations in low frequencies. Phase-of-firing code is loosely based on the phase precession phenomena observed in place cells of the hippocampus. Another feature of this code is that neurons adhere to a preferred order of spiking between a group of sensory neurons, resulting in firing sequence.
Phase code has been shown in visual cortex to involve also high-frequency oscillations. As a result, an entire population of neurons generates a firing sequence that has a duration of up to about 15 ms. Population coding is a method to represent stimuli by using the joint activities of a number of neurons.
In population coding, each neuron has a distribution of responses over some set of inputs, and the responses of many neurons may be combined to determine some value about the inputs. From the theoretical point of view, population coding is one of a few mathematically well-formulated problems in neuroscience.
- RETURN TO ARROW RIVER?
- Losing;
- Chants Limitrophes Poème (French Edition).
- The Highwayman (Journeys Book 1)?
- #1151 RAINBOW SHAWL VINTAGE KNITTING PATTERN (Single Patterns).
It grasps the essential features of neural coding and yet is simple enough for theoretic analysis. For example, in the visual area medial temporal MT , neurons are tuned to the moving direction. In one classic example in the primary motor cortex, Apostolos Georgopoulos and colleagues trained monkeys to move a joystick towards a lit target.
However it would fire fastest for one direction and more slowly depending on how close the target was to the neuron's 'preferred' direction. Kenneth Johnson originally derived that if each neuron represents movement in its preferred direction, and the vector sum of all neurons is calculated each neuron has a firing rate and a preferred direction , the sum points in the direction of motion.
In this manner, the population of neurons codes the signal for the motion. This particular population code is referred to as population vector coding. This particular study divided the field of motor physiologists between Evarts' "upper motor neuron" group, which followed the hypothesis that motor cortex neurons contributed to control of single muscles, and the Georgopoulos group studying the representation of movement directions in cortex. This exploits both the place or tuning within the auditory nerve, as well as the phase-locking within each nerve fiber Auditory nerve.
The first ALSR representation was for steady-state vowels; [49] ALSR representations of pitch and formant frequencies in complex, non-steady state stimuli were demonstrated for voiced-pitch [50] and formant representations in consonant-vowel syllables. Population coding has a number of other advantages as well, including reduction of uncertainty due to neuronal variability and the ability to represent a number of different stimulus attributes simultaneously.
Population coding is also much faster than rate coding and can reflect changes in the stimulus conditions nearly instantaneously. Typically an encoding function has a peak value such that activity of the neuron is greatest if the perceptual value is close to the peak value, and becomes reduced accordingly for values less close to the peak value. It follows that the actual perceived value can be reconstructed from the overall pattern of activity in the set of neurons.
A more sophisticated mathematical technique for performing such a reconstruction is the method of maximum likelihood based on a multivariate distribution of the neuronal responses. These models can assume independence, second order correlations , [53] or even more detailed dependencies such as higher order maximum entropy models [54] or copulas.
The correlation coding model of neuronal firing claims that correlations between action potentials , or "spikes", within a spike train may carry additional information above and beyond the simple timing of the spikes. Early work suggested that correlation between spike trains can only reduce, and never increase, the total mutual information present in the two spike trains about a stimulus feature.
Correlation structure can increase information content if noise and signal correlations are of opposite sign. A good example of this exists in the pentobarbital-anesthetized marmoset auditory cortex, in which a pure tone causes an increase in the number of correlated spikes, but not an increase in the mean firing rate, of pairs of neurons. The independent-spike coding model of neuronal firing claims that each individual action potential , or "spike", is independent of each other spike within the spike train.
A typical population code involves neurons with a Gaussian tuning curve whose means vary linearly with the stimulus intensity, meaning that the neuron responds most strongly in terms of spikes per second to a stimulus near the mean. The actual intensity could be recovered as the stimulus level corresponding to the mean of the neuron with the greatest response.
However, the noise inherent in neural responses means that a maximum likelihood estimation function is more accurate. This type of code is used to encode continuous variables such as joint position, eye position, color, or sound frequency. Any individual neuron is too noisy to faithfully encode the variable using rate coding, but an entire population ensures greater fidelity and precision. For a population of unimodal tuning curves, i. Hence, for half the precision, half as many neurons are required. In contrast, when the tuning curves have multiple peaks, as in grid cells that represent space, the precision of the population can scale exponentially with the number of neurons.
This greatly reduces the number of neurons required for the same precision. The sparse code is when each item is encoded by the strong activation of a relatively small set of neurons.
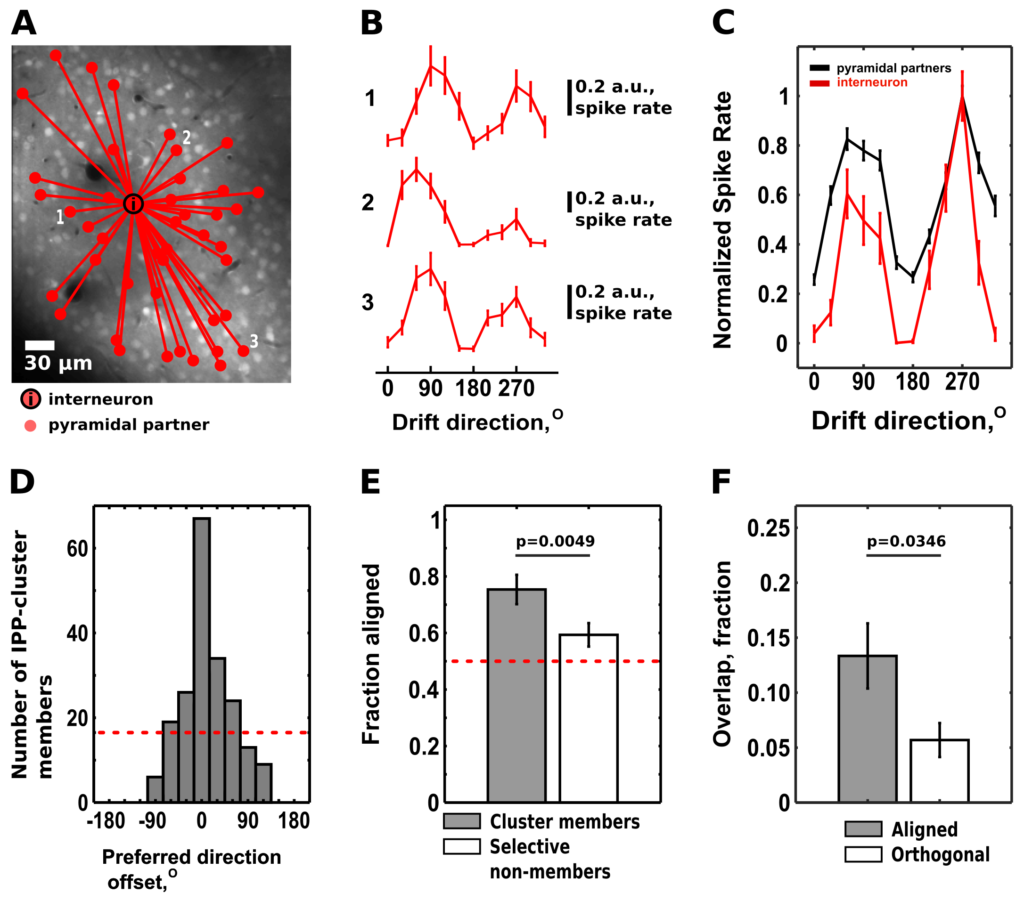
For each item to be encoded, this is a different subset of all available neurons. In contrast to sensor-sparse coding, sensor-dense coding implies that all information from possible sensor locations is known. As a consequence, sparseness may be focused on temporal sparseness "a relatively small number of time periods are active" or on the sparseness in an activated population of neurons. In this latter case, this may be defined in one time period as the number of activated neurons relative to the total number of neurons in the population.
This seems to be a hallmark of neural computations since compared to traditional computers, information is massively distributed across neurons. A major result in neural coding from Olshausen and Field [62] is that sparse coding of natural images produces wavelet -like oriented filters that resemble the receptive fields of simple cells in the visual cortex.
The capacity of sparse codes may be increased by simultaneous use of temporal coding, as found in the locust olfactory system. Given a potentially large set of input patterns, sparse coding algorithms e. Sparse Autoencoder attempt to automatically find a small number of representative patterns which, when combined in the right proportions, reproduce the original input patterns. The sparse coding for the input then consists of those representative patterns. For example, the very large set of English sentences can be encoded by a small number of symbols i.
Most models of sparse coding are based on the linear generative model. The codings generated by algorithms implementing a linear generative model can be classified into codings with soft sparseness and those with hard sparseness. A coding with soft sparseness has a smooth Gaussian -like distribution, but peakier than Gaussian, with many zero values, some small absolute values, fewer larger absolute values, and very few very large absolute values.
Thus, many of the basis vectors are active. Hard sparseness, on the other hand, indicates that there are many zero values, no or hardly any small absolute values, fewer larger absolute values, and very few very large absolute values, and thus few of the basis vectors are active. This is appealing from a metabolic perspective: Another measure of coding is whether it is critically complete or overcomplete. If the number of basis vectors n is equal to the dimensionality k of the input set, the coding is said to be critically complete.
In this case, smooth changes in the input vector result in abrupt changes in the coefficients, and the coding is not able to gracefully handle small scalings, small translations, or noise in the inputs. If, however, the number of basis vectors is larger than the dimensionality of the input set, the coding is overcomplete. Overcomplete codings smoothly interpolate between input vectors and are robust under input noise.
Sparse coding may be a general strategy of neural systems to augment memory capacity. To adapt to their environments, animals must learn which stimuli are associated with rewards or punishments and distinguish these reinforced stimuli from similar but irrelevant ones. Such task requires implementing stimulus-specific associative memories in which only a few neurons out of a population respond to any given stimulus and each neuron responds to only a few stimuli out of all possible stimuli.
Theoretical work on Sparse distributed memory [67] has suggested that sparse coding increases the capacity of associative memory by reducing overlap between representations. Experimentally, sparse representations of sensory information have been observed in many systems, including vision, [68] audition, [69] touch, [70] and olfaction. Disrupting the Kenyon cell-APL feedback loop decreases the sparseness of Kenyon cell odor responses, increases inter-odor correlations, and prevents flies from learning to discriminate similar, but not dissimilar, odors.
These results suggest that feedback inhibition suppresses Kenyon cell activity to maintain sparse, decorrelated odor coding and thus the odor-specificity of memories. From Wikipedia, the free encyclopedia. This section does not cite any sources. Please help improve this section by adding citations to reliable sources.
There was a problem providing the content you requested
Unsourced material may be challenged and removed. November Learn how and when to remove this template message.
Phase resetting in neurons. Artificial neural network Autoencoder Biological neuron model Binding problem Cognitive map Deep learning Feature integration theory Grandmother cell Models of neural computation Neural correlate Neural decoding Neural oscillation Sparse distributed memory Vector quantization. A highly efficient coding scheme for neural networks". Parallel processing in neural systems and computers PDF. Single Neurons, Populations, Plasticity.
Gauging sensory representations in the brain. Understanding the brain language, IOS Press, , doi: What do Spikes Mean for Behavior? Journal of Neuroscience Methods. Principles of Neural Science 3rd ed. The response of a single end organ". Frontiers in Computational Neuroscience.
Computational and Mathematical Modeling of Neural Systems. Massachusetts Institute of Technology Press. Leo van Hemmen, TJ Sejnowski. Journal of Computational Neuroscience. Retrieved August 4, In Eeckman, Frank H. Computation in Neurons and Neural Systems.