Bayesian Computation with R (Use R!)
If for any reason your order is not available to ship, you will not be charged.
Popular Searches
Your order is also backed by our In-Stock Guarantee! What makes Biblio different?
- Introduction.
- The Sock Problem?
- From Geordie Land to No Mans Land!
Sign In Register Help Cart. Search Results Results 1 -9 of 9. Bayesian Computation with R Use R!
Free e-Copy of Bayesian Computation with R (Use R)
All text is legible and may contain extensive markings, highlighting, worn corners, folded pages, etc. Ergodebooks , Texas, United States Seller rating: Ships with Tracking Number! May not contain Access Codes or Supplements.
Buy with confidence, excellent customer service! We're sorry - this copy is no longer available. More tools Find sellers with multiple copies Add to want list.
- The Synthesis of Three Dimensional Haptic Textures: Geometry, Control, and Psychophysics (Springer Series on Touch and Haptic Systems)?
- Approximate Bayesian Computation.
- Daughters of the Sea #3: Lucy!
- I Was Made to Love Her!
- Connor & James and The Case of the Beetle Stone.
- EROTIC POETRY: Explicit Thoughts From Jersey Citys Finest.
- Special offers and product promotions;
Didn't find what you're looking for? Add to want list. Are you a frequent reader or book collector?
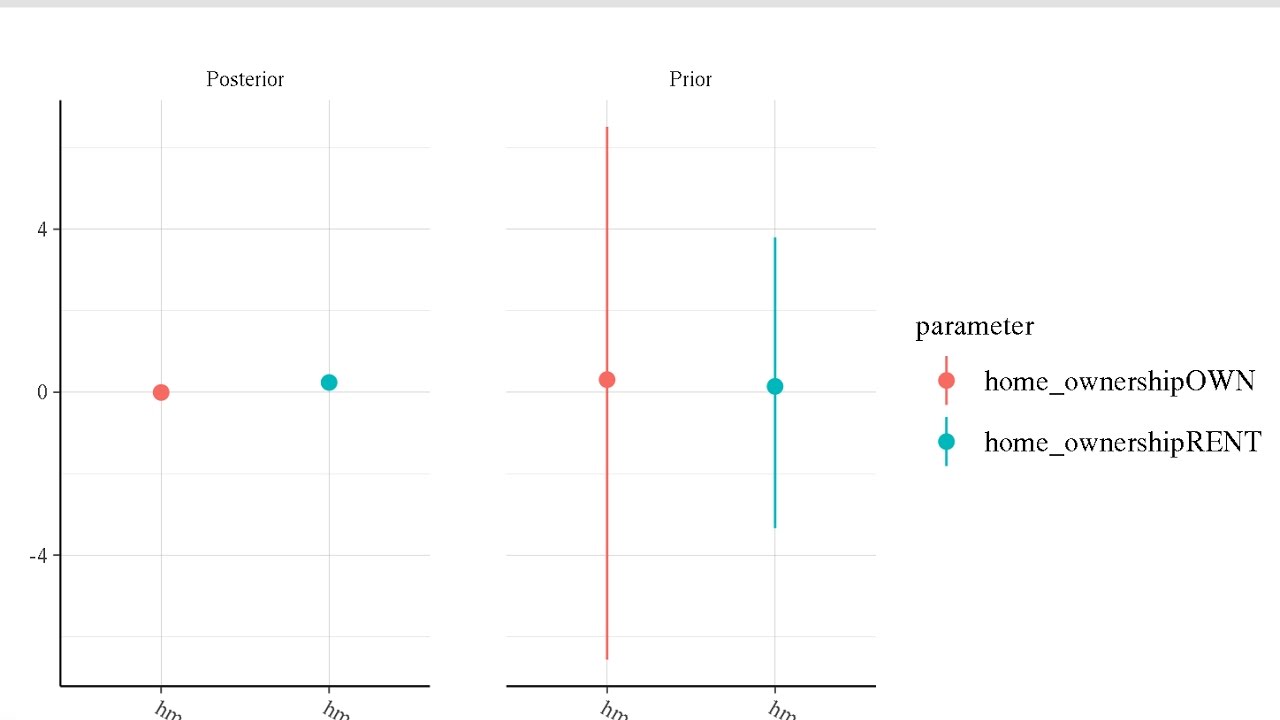
Social responsibility Did you know that since , Biblio has used its profits to build 12 public libraries in rural villages of South America? Assume prior probability distributions over all the parameters that you want to estimate. Sample tentative parameters values from the prior distributions, plug these into the generative model and simulate a dataset. Check if the simulated dataset matches the actual data you are trying to model.
Bayesian Methods UCSD
If yes, add the tentative parameter values to a list of retained probable parameter values, if no, throw them away. Repeat step 2 and 3 a large number of times building up the list of probable parameter values. Finally, the distribution of the probable parameter values represents the posterior information regarding the parameters.
Intuitively, the more likely it is that a set of parameters generated data identical to the observed, the more likely it is that that combination of parameters ended up being retained. The Sock Problem The problem is this: If you know the parameters and the data, this is the way we generate a sample: Assuming than an average person washes, each time, 30 socks and giving a confortable standard deviation of 15, we have: We select a Beta for the job: The true answer for the sock problem was: